In this article, we examine how attribution has transformed from a purely technical exercise into a strategic framework for decision-making, while grappling with the challenges posed by increasing automation.
What is Attribution? #
Attribution is the act of assigning credit to marketing channels (touchpoints), while an attribution model is the rule or algorithm that determines how the credit is distributed among them. Marketing Attribution is the overall process of determining which marketing touchpoints are contributing to desired outcomes (like conversions, leads, or sales). It includes collecting data, applying attribution models, analyzing the results and using insights to improve marketing strategies.
The action (attribution), the method (attribution model), and the overall process (marketing attribution).
The Complexity of Attribution #
Seasoned marketing professionals have long grappled with the complexities of attribution.
The complexity of attribution lies in its dual nature as both a practical tool for evaluating marketing performance and a conceptual framework for decision-making.
At its core, attribution is about understanding the impact of various marketing strategies on outcomes. However, it goes beyond being merely a model or algorithm; it transcends these technical aspects to become a conceptual framework for defining success within the context of a brand and identifying the key factors that drive it.
As Measurement Tool: Quantifies each dollar’s origin- Quantifies channel contributions to revenue
- Determines which channels get future budgets
This duality highlights that regardless of the method used — whether it involves machine learning or a simple spreadsheet — the financial outcome (revenue or conversions) remains unchanged if the calculations are correct. What does change, however, is the interpretation of those outcomes, which is based on how different methods assign credit to various touchpoints along the customer journey.
In that sense, attribution modeling is more than a technical exercise — it’s a strategic lens through which we interpret data to:
- Define success
- Guide budget allocation
- Identify winning strategies
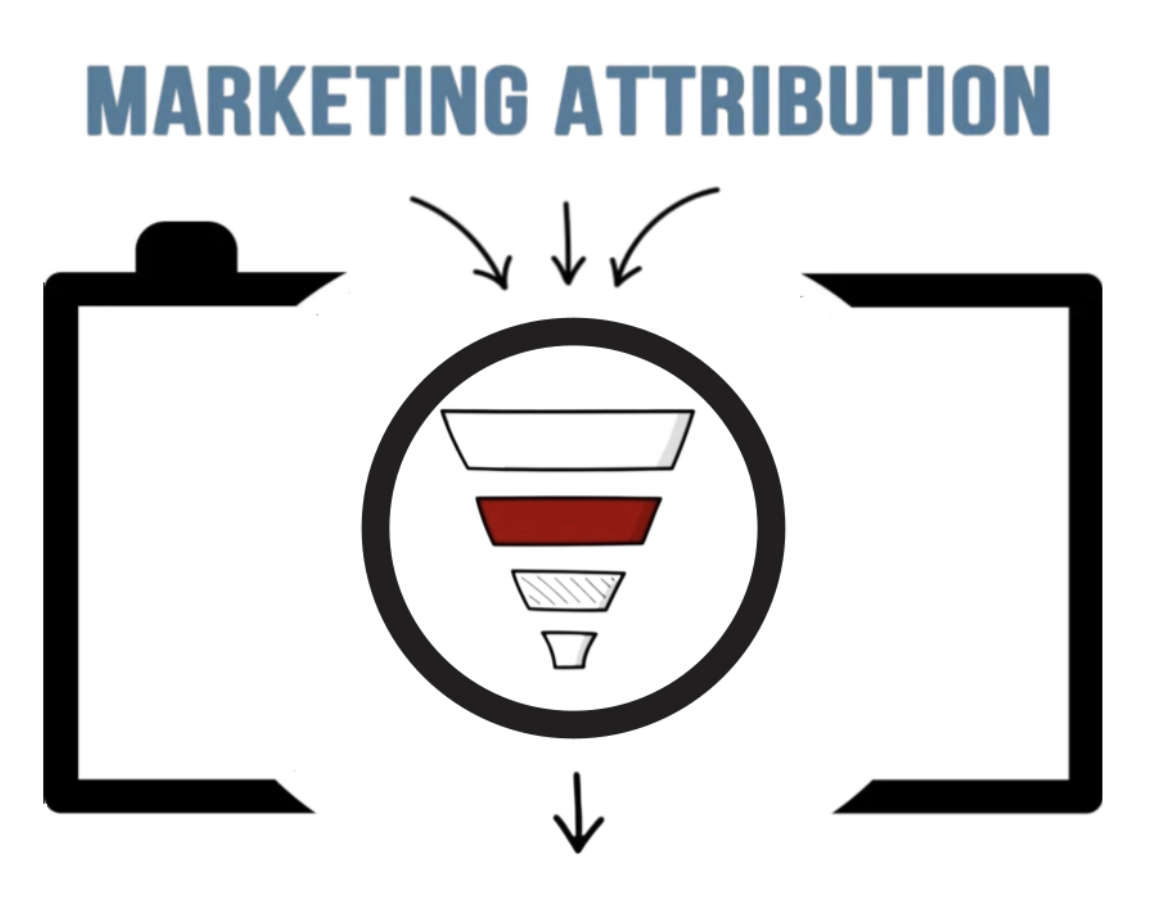
Attribution as a Lens of Opinion #
Attribution is not about finding the “truth” but about gaining a clearer perspective.
Attribution models should be viewed as different lenses through which to analyze marketing performance. Each model tells a story based on the rules applied, and different models may be more appropriate for different scenarios. For instance, last-click attribution might not be suitable for prospecting campaigns, while first-touch attribution might not work well for direct response activities.
The models we use are just that — models. They are not definitive answers but tools in our analytical toolkit to view data through different lenses. Everyone is technically correct in their interpretations as long as they are based on the same accurate data.
This understanding has transformed how we approach data. We no longer search for one ‘correct’ answer. Instead, we recognize these models as opinions or perspectives. Recognizing that these models are subjective allows us to choose the most appropriate one for each situation without being constrained by the pursuit of a one-size-fits-all solution.
Use attribution for the value it brings, not to get stuck in endless analysis.
The real value of attribution lies in its ability to transform raw data into meaningful insights. That means choosing a model that’s understandable, explainable, and actionable. Even if you use a basic model like last-click attribution, doing so with an awareness of its limitations allows you to make informed decisions.
“The goal isn’t perfect attribution — it’s making better decisions with the information you have.” – Tom Shapiro, CEO of Stratabeat
After all, having a perfect model without actionable decisions is less valuable than having an imperfect model that drives some level of decision-making.
The Importance of Attribution #
The significance of attribution mainly depends on two key factors: the complexity of your customer journey and the nature of your product or service.
Low-Value, Single-Touch Products:
If you’re selling low-ticket items, single-touch products like batteries on Amazon, where purchases are often impulsive and involve minimal interaction, attribution might be lower in importance.
In such cases, the customer journey is straightforward, and attribution models won’t provide much additional value since the decision-making process is simple and direct.
High-Value, Multi-Touch Products:
However, if you’re selling high-ticket items like $20,000 cruises, where the customer journey is complex and involves multiple touchpoints — across different campaigns, channels, or even organic interactions — attribution becomes far more critical.
In this scenario, understanding how different keywords, campaigns, and interactions contribute to the final conversion is essential.
The Role of Attribution #
Attribution in marketing serves multiple purposes, both strategic and justificatory.
Attribution is inherently backward-looking. So, it might appear as a retrospective tool used to justify past spending. However, attribution can also be a proactive tool to inform future spending decisions.
Attribution can indeed be historical, analyzing past data to evaluate the effectiveness of different marketing activities. This is particularly useful in industries with long sales cycles, where it may take several months to see the results of marketing efforts. In such cases, looking back over a substantial period (like nine months) is necessary to gain a complete understanding of which activities eventually led to conversions.
On the other hand, its true value lies in providing insights that help marketers make informed budget allocations for future campaigns. For example, in planning a major campaign like a Black Friday sale, marketers can use data from previous years to forecast outcomes and allocate budgets more effectively across various channels or customer segments.
Moreover, attribution helps in refining the understanding of customer interactions across different stages of the sales funnel—top, middle, and lower funnel. Without a robust attribution model, businesses might rely too heavily on simplistic metrics like last-click attribution, which often biases budget allocation towards activities that close sales (lower funnel) rather than those that initiate customer interest (top of the funnel).
Attribution Doesn’t Measure Incrementality
Attribution has limitations, especially when it comes to measuring incrementality — it can’t determine if a conversion would have happened without a specific marketing activity. Since attribution models only analyze visible touchpoints and assign credit based on predefined rules (e.g., last-click, linear, data-driven), they don’t fully answer the question: ““Would this conversion have happened anyway?”.
To measure incrementality, additional techniques are needed, such as:
- Randomized Control Trials (RCTs): Compare results between exposed and unexposed groups.
- Geo-Based Testing: Analyze performance differences across regions with varying ad spend.
- Media Mix Modeling (MMM): Use statistical analysis to measure the impact of different marketing channels at a macro level.
The Challenges of Attribution #
Up until now, we’ve primarily discussed attribution through the lens of deterministic measurement — where every touchpoint in a customer’s journey can be precisely measured and analyzed with sufficiently high data quality to make sense of it. However, when we introduce factors like data anonymization, missing data due to privacy regulations or technologies, or fragmented customer journeys across unconnected mobile apps where users aren’t logged in, the picture becomes more complex.
This brings up the question about the phenomenon known as diminishing return on deterministic attribution and the growing role of media mix modeling (MMM) and data modeling in addressing these challenges.
Media Mix Modeling #
The question arises:
At what point should a brand consider media mix modeling (MMM) solutions instead of solely relying on deterministic methods?
Deterministic attribution thrives in environments where data is abundant, high-quality, and easily trackable.
So, the first step is to thoroughly understand the quality and integrity of your data. While you’ll never know what you don’t know, it’s crucial to be aware of the limitations and scope of the data you have. This means entering the process with a clear understanding of the “playground” you’re operating in and the data available to you.
For brands investing heavily in non-trackable activities—such as traditional TV (not connected TV, though some tracking is possible there), outdoor advertising, print, and similar channels—media mix modeling undoubtedly plays a role. These channels are far less trackable compared to digital spaces, where we have significantly more data. Combining media mix modeling with machine learning-based attribution models for digital channels can provide a more holistic view.
The key principle here isn’t to track every single interaction meticulously but rather to facilitate smarter decision with the information you have. If we can improve decision-making with the data we have, we’re moving in the right direction.
Data Modeling #
Platforms like Google Analytics and other marketing tools are increasingly incorporating data modeling capabilities, using machine learning to estimate missing data. While this approach can fill gaps and provide actionable insights, it also raises concerns about transparency and reliability.
Perhaps the most significant concern in this evolution is the potential for uncritical acceptance of automated results. For many marketers, especially those new to the field or less involved in analytics, the automated approach might seem like a welcome relief. However, this convenience comes at the potential cost of critical thinking and strategic insight.
The key is to strike a balance. Modeled data can be incredibly useful when used in conjunction with human judgment and critical thinking. However, over-reliance on automated models risks creating a “set it and forget it” mentality, where marketers accept results without questioning their validity.
Conclusion #
As automation and data complexity grow, the debate around attribution continues, but one thing is remain constant: attribution is no just about numbers; it’s about understanding the story behind the data and using it to drive meaningful action.